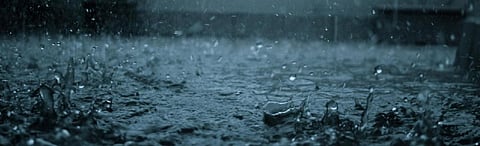
There were 354 heavy rainfall events — defined by IMD as absolute rainfall above 64.5 millimetres (mm) — across India in March and April.
Frequency of rainfall in March 2020 (category: >64.4 mm)
Source: Giriraj Amarnath/IWMI
Frequency of rainfall in April 2020 (category: >64.4 mm)
Source: Giriraj Amarnath/IWMI
Of these events, 224 occurred in April, while 130 occurred in March, according to an analysis by Down To Earth and Colombo-based research institute International Water Management Institute (IWMI).
Jammu and Kashmir and Ladakh were impacted the most (52 occurrences), followed by West Bengal (41) and Odisha (40), while Meghalaya recorded 29 such events in two months.
The heavy rain and storms led to cooler-than-normal temperatures across north, central and east India.
IMD predictions
The maximum temperatures recorded by IMD were below normal in large parts of north, east, central and southern parts of the country as recent as on May 11, according to the latest data available. Temperatures were lower by 4-7 degrees Celsius (°C), in parts of Uttar Pradesh, Bihar and Jharkhand.
“Warming of land surface in the summer, especially in the northern part of the country, is essential for attracting monsoon winds that occur due to temperature contrasts between land and ocean,” said Raghu Murtugudde, a climate scientist at the University of Maryland in the United States (US).
The coming south-west monsoon may, thus, be weakened as a result of a cooler-than-normal north and central India during the pre-monsoon period.
This was in stark contrast with the rest of the world, with a warmer-than-usual 2020.
The global average land and ocean surface temperature for March stood at 1.6 °C, above the 20th century average of 12.7 °C, according to the US National Centers for Environmental Information and the National Aeronautical and Space Administration.
IMD also predicted warmer-than-usual weather for the months of March and April for India. It said average maximum temperatures were likely to be warmer-than-usual by 0.5-1°C for April, May and June in its latest seasonal outlook for temperatures on April 1.
The IMD made a similar prediction for the months of March, April and May as well, but temperatures were below normal in large parts of the country for March, April and now May. There were no heat waves recorded in the country through May 13.
Western disturbances
The main reason for storms, rainfall and cooler temperatures were western disturbances: Extra-tropical storms that originate in the Mediterranean and travel to India on the sub-tropical jet stream.
Frequent western disturbances could be the result of “unusually warm temperature over Eurasia and the warm-cool pattern over the Atlantic Ocean working together to favour these western disturbances during the pre-monsoon”, according to Murtugudde.
“In general, they (western disturbances) would bring dust storms, but in this case, they probably brought enough moisture. So, when they met warm air over western India, they produced thunderstorms and rain,” he added.
It was difficult for the IMD to foresee western disturbances as they are often difficult to predict.
Moisture is carried in the upper atmosphere unlike tropical storms that pick up moisture from the surface, said Murtugudde.
The western disturbance can only be tracked and predicted well around a day in advance, as they were cyclonic systems riding on the sub-tropical jet stream, according to him.
The IMD usually does well in predicting weather phenomena that have larger scales, like high temperatures and heat waves, rather than smaller phenomena like storms that bring hail, dust and lightning that occurred in March and April, said Murtugudde.
He pointed out a major loophole in the IMD’s prediction for the south-west monsoon, a major weather phenomenon for the country that brings major rainfall.
The models used by the IMD also depended too heavily on El Nino Southern Oscillation and Indian Ocean Dipole global climatic phenomena, while ignoring the Atlantic Niño or the Atlantic Zone Mode, he said.
This Atlantic mode was done wrong in the Coupled Forecasting System model used by the IMD, affecting forecasts, he added, citing a paper by the Indian Institute of Tropical Meteorology based out of Pune, Maharashtra.
A warmer Atlantic led to fewer monsoon depressions bringing more rain, while a colder Atlantic led to more monsoon depressions, according to a study published in the Journal of Geophysical Research, a scientific journal, in 2014.
Depressions during the warm Atlantic mode had longer tracks, leading to better distribution of rain over the Indian subcontinent, the study said.
It also showed a tele-connection link between the conditions in the Atlantic and Indian Oceans, crucial for understanding and forecasting monsoon behaviour.
This is when it becomes important for the IMD to get its forecasts right. It is the only public meteorological organisation in the country with a vast network of weather observatories and instruments and the technical ability to make credible forecasts.
There are some issues, however, with certain aspects of data collection and coordination between the IMD — that comes under the Union government — and state governments and private entities that also collect weather-related information, but cannot make their own forecasts.
Automatic weather stations
One issue is with the density of the observation network: The Union government contends the country needed around 40,000 automatic weather stations (AWS) with a spacing of 10 km in the plains and 5 km in the hills.
An AWS collects data on rainfall, temperature and humidity wind direction, wind speed, atmospheric pressure and solar radiation.
India, however, currently has only a third of the required number, according to a report released by Delhi-based non-profit Centre for Science and Environment (CSE).
These AWSs are owned by both: Governments (Union and state) and private companies. IMD owns 700 of these AWSs.
There is also a drastic difference in the scale of weather data collection infrastructure across states. In Kerala, there is one AWS for every 87 square km, while in Assam there is one in every 472 square km.
For some states — including Punjab, Haryana, Uttar Pradesh and Chhattisgarh — this number cannot be correctly predicted, as the number of state-owned weather stations remains unknown.
The data collected from these AWSs is also not standardised, which means they will produce inaccurate predictions when fed into a climate modelling system.
“This lack of standardisation arises from the fact that the recent and ongoing expansion in the AWS network has proceeded with minimal coordination and oversight,” the CSE report pointed out.
It said that this occurred as AWSs were mostly installed in a project mode “with a focus on hitting short-term targets within a particular area or institution”.
Not much importance was given to how such AWS network creation / expansion ‘projects’ link to a larger weather data system, resulting in a lack of good quality data for modelling.
The quality of data coming from AWSs is poor as well, due to a lack of quality control of weather stations, no proper maintenance and absence of a common platform for data collation from different sources.
A possible underlying cause is that maintenance is based on hiring a qualified local resident. The budget to sustain such a hire is often unavailable once the ‘project’ (that is, installing the station / gauge) is considered complete.
“The result is a fundamental confusion regarding the exact extent of the ‘operational’ network (as opposed to the ‘installed’ network),” said the report.
Data assimilation techniques can help plug data density and quality gaps, but only to an extent.
It is difficult to generate forecasts at a block or village level based on district level data, while keeping information reliable and giving enough time for officials on ground to take necessary action during a calamity, like a flood or cyclone.